Building a recommendation system using AI
About client
Our client is an international media organization with over 28 years of rich history. They have their presence in USA, Europe, Asia, Africa and Australia.
The Client broadcasts inspirational content on their platform. Their platform allows publishing of even those content which are independently produced. All of their content is intended to uplift, encourage and assist viewers.
Requirement
Collect user data, to comprehend the data and to profile the users based on their choices on viewing the content.
And, for this, client wanted us to build an AI Recommendation Engine which can help them collect user data and understand it. By doing this, client wanted to cater to the specific needs of the users, intending to provide content that will aid in the growth of the user.
What is AI based Recommendation Engine and how does it help?
AI enables recommendation engines to quickly and to-the point tailor each customer’s recommendations to their requirements and preferences. Online searching is becoming more effective thanks to AI because it now generates suggestions based on the customers’ visual preferences rather than product details. AI-powered recommendation engines assist users in finding items or material that they might not otherwise notice. Because of this, recommendation engines are crucial to the success of companies like Amazon, Facebook, YouTube, etc.

For anticipating the needs of a particular user, our recommender system considers the following:
- Previously viewed videos/history
- Liked videos
- Favourite/saved Videos
- Past purchases
- Most watched and watched full length videos
- Factors such as Genre, Speaker, Type of video, Topic
- User's profile (where age, gender, profession, hobbies, spiritual level are indicated)
- Similar profiles of other users
- Geographic location
The primary goal of all AI search strategies is to enhance the comprehension of long, complicated queries and deliver the right answer even when the input data is inaccurate or partial. Systems without AI uses simple algorithms which makes expected recommendation predictive and limited.
Challenges
- Without high quality data, or without crunch and proper analysis, leads recommendation engine to perform poorly
- To collect explicit and implicit data of users which is whether they liked the content or not and other information
- To profile users detail based on their viewing pattern
- To choose the right recommendation engine which will give the desired output
- To set algorithms for fast and to-the point content predictions/recommendation
After testing various AI based algorithms and filtering systems such as Item based, User Based, Content Based, Collaborative, Hybrid filtering models, team concluded that Hybrid Filtering system is the best solution for our recommendation system.
Solution
- Hybrid filtering algorithm combines both Content and Collaborative filtering with AI recommendations
- Rather than using specific queries, AI recommendation systems evaluate user preferences and recommend desirable output
- Capture login data of registered users(new and old) and guest users
- Collect user data based on their viewing pattern and profile them accordingly
- Capture implicit and explicit user data for understanding and recommendation
- Faster and to-the point recommendations
- Narrow down searches to specific items reducing the time it takes to find desired products and services
- Significantly increase the likelihood of other potentially appealing videos getting into the user’s field of vision
- Encourage users to interact with more products I.e; genres, videos, speakers etc, and this leads to increased consumption and increased profits
Hybrid Recommendation System
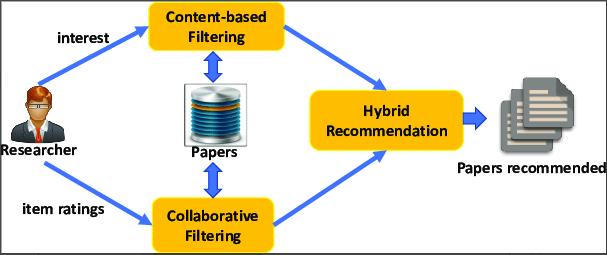
Technologies and tools
Python v3.10, MongoDB v3.6, PHP v7.4, MySql v10, HTML, Bootstrap v4.3
Benefits to the Client
- With fast prediction and tailor made content, it boosts visit frequency of users leading to increase in potential lead data
- Contributes to higher view/purchase rates increasing customer loyalty and profit
- Decreases customer churn leading to rise in recurrent revenue and customer base
- Decrease in marketing cost with the use of tailored content, newsletters, personalized ads, and push notifications while encouraging users to keep
- coming back
- Increase in personalized and compelling content with fast and factual predictions leaving users satisfied
- Increase search listings down to user-specific items, thereby reducing the time needed to find desired content
- The system will not only show similar items liked by a particular user but also similar type of items liked by other users
- Contribute to a higher viewer/purchase rate, user loyalty, and satisfaction with web services increases
- Newsletters, personalized advertisements, and push notifications to encourage users to come back, increase the frequency of visits by repeat users,
- and reduce customer churn
